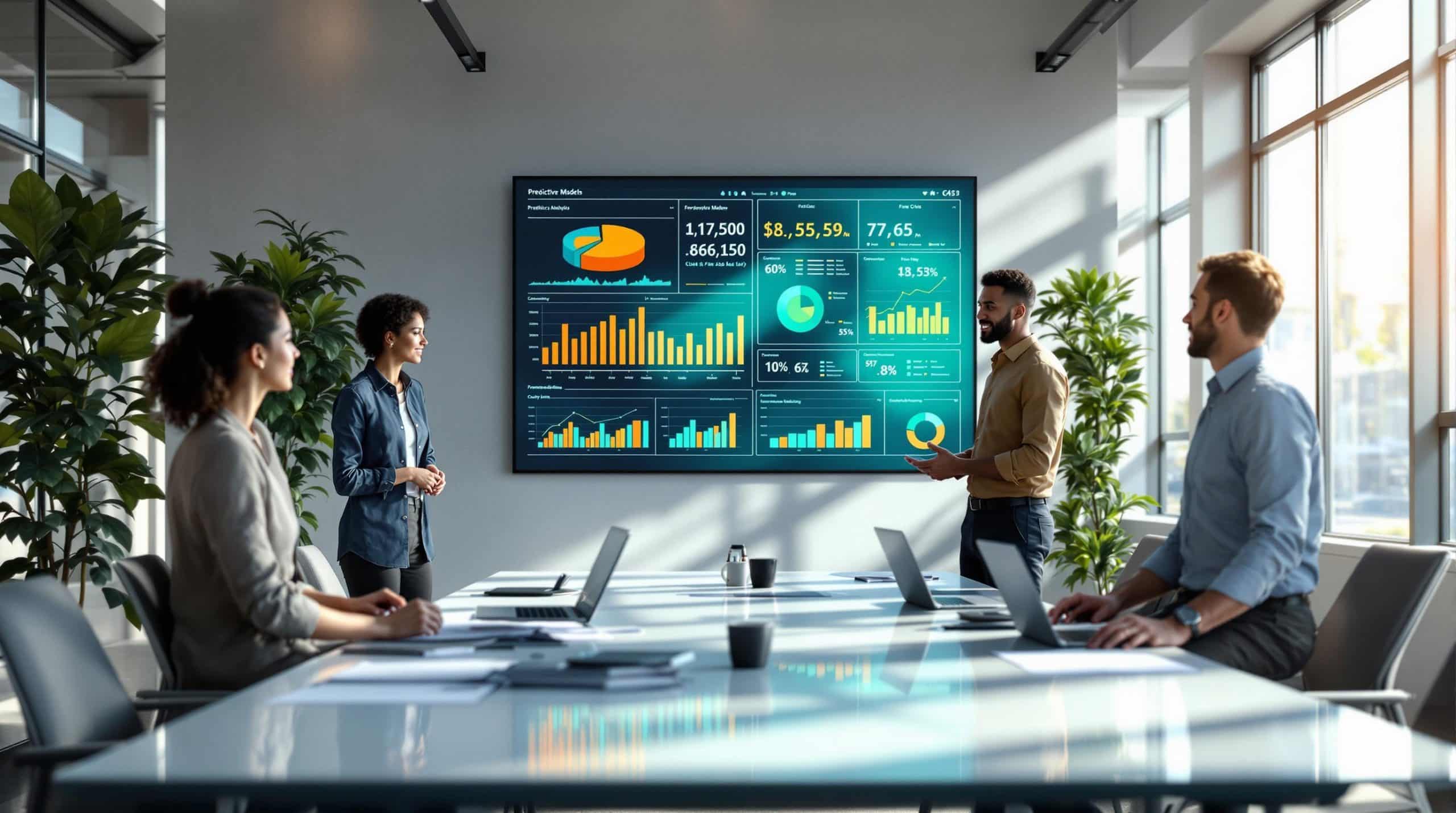
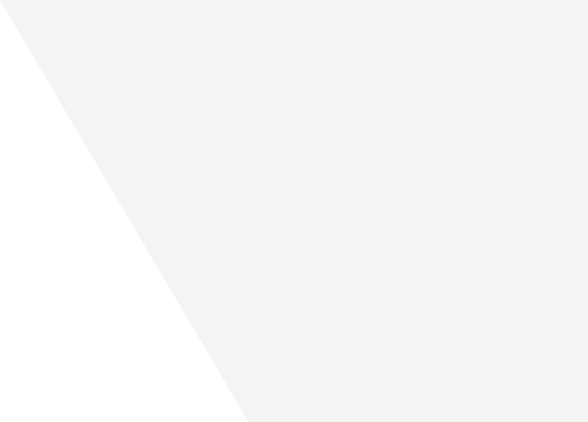
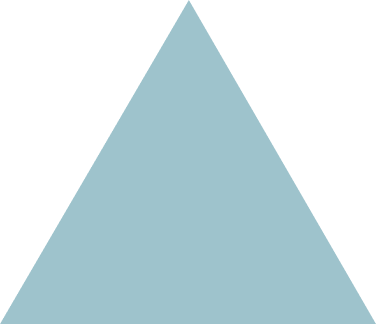
Predictive models help create more accurate cost estimates by analyzing market trends and various factors simultaneously. These factors include:
By recognizing patterns, these models can flag potential cost overruns early, allowing teams to address issues during the tender process. This leads to better planning and allocation of resources.
Different tender needs call for different types of predictive models:
Once you’ve chosen the right model, the next step is integrating it into your existing systems.
Assessment Phase Start by evaluating your current tender processes. Identify key decision points where predictive models can add value and make adjustments to workflows as needed.
Implementation Strategy
System Integration Connect predictive models to your existing software for smooth data flow and reliability. A pilot project can help validate the integration before scaling up.
For infrastructure projects, consider lifecycle cost modeling to uncover savings throughout the project’s duration. Companies that use predictive models during the tender process often achieve more precise cost estimates and better resource management.
Infrastructure projects have shown how predictive modeling can help reduce tender costs. For example, in a bridge maintenance contract, optimizing resource allocation and maintenance schedules led to noticeable cost savings. Similarly, a highway expansion project used better forecasting to estimate maintenance needs and material quantities, resulting in more competitive bids and tighter budget control.
These examples highlight clear improvements in scheduling, resource use, and bid accuracy, paving the way for more tailored strategies to fine-tune tender cost management.
Oxand uses historical data and probabilistic models to fine-tune maintenance schedules and tender pricing strategies. Their database, which includes over 10,000 proprietary predictive models, helps infrastructure managers achieve:
One major infrastructure operator successfully applied these predictive models to determine the best maintenance intervals and resource needs. This data-driven method not only enhanced their tender competitiveness but also ensured service quality remained high. For long-term infrastructure contracts, combining historical data with probabilistic analysis has proven especially effective in predicting lifecycle costs with precision.
Predictive models often struggle when data quality is poor. Issues like incomplete records, inconsistent formats, or scattered data sources can disrupt their effectiveness.
Here’s how to tackle these challenges:
With clean, reliable data in place, the next step is ensuring teams work together effectively.
Introducing predictive models to established tender processes can face pushback from team members resistant to change.
To build collaboration and trust:
Once teamwork is on track, keeping the models updated becomes the priority.
Over time, the accuracy of predictive models can drop as markets, regulations, and cost structures shift. Regular updates are critical to maintaining their relevance and reliability.
Key practices include:
For infrastructure projects, it’s especially important to adjust for evolving maintenance needs and asset conditions. Regular calibration ensures cost forecasts stay dependable throughout the tender process.
Predictive models play a key role in managing tender costs by enabling smarter, data-driven decisions. Companies using these tools often see reduced costs and improved resource management.
Oxand’s approach highlights how predictive modeling can reshape tender planning and execution. Their methods show how structured modeling can make a real difference in project outcomes, proving the importance of including predictive tools in tender strategies.
Success in this area depends on three main factors:
These elements form the foundation of effective tender cost management.
Beyond cutting costs, predictive modeling supports smarter asset lifecycle planning. By analyzing past data and forecasting strategically, organizations can better prepare for future demands while making the most of their current resources.
Adopting predictive modeling not only gives companies an edge in tender processes but also helps build more efficient, data-driven operations for long-term success.